Introducing AI into Electricity Load Forecasting: Deep Learning Optimizes Power Plants’ Operation
2019/11/13 Toshiba Clip Team
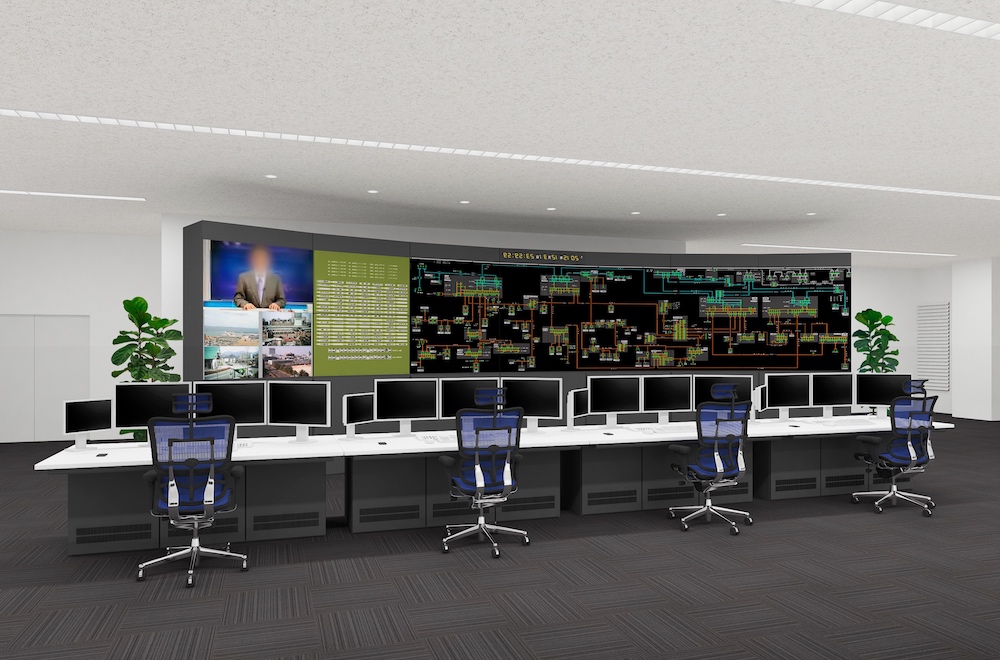
In 2017, Toshiba Corporate R&D Center’s system engineering laboratory won the top prize in the 1st Electricity Load Forecasting Technology Contest organized by Tokyo Electric Power Company Holdings Inc. (TEPCO). The contest covered nine days in early September, considered a season where demand was hard to predict. Contestants received electricity load data up to the day before the period and then had to predict demand for the following day in one-hour units. They were then evaluated on the precision of their results.
In this article, we shine a spotlight on Toshiba’s load forecasting system that beat over 100 other entries from around the world to win the top prize.
New electric power and renewable energies means more need for electricity load forecasting
Electric power providers carry out electricity load forecasts so that they can draw up their daily supply and transaction plans. There is a principle in the electric power industry known as “the balancing rule.” In other words, the amount required must be supplied at the time that it is required, and there must always be a consistent equal balance between the amount demanded and the amount supplied. Any excess operation by a power plant or undersupply of electric power leads to loss for the operator, so producing just enough to cover demand is the ideal situation. The direct trigger that prompted TEPCO to hold the first-ever Electricity Load Forecasting Technology Contest in 2017 was most likely the liberalization of electric power generation in Japan in 2016. Greater liberalization led to an increase in small-scale providers known as “new electric power” and a massive adoption of renewable energies, mainly solar power. This in turn made it harder to formulate efficient supply plans, resulting in a sudden, greater need for high-precision load forecasting technology.
Electrical loads were traditionally predicated manually based on accumulated past records, weather phenomena, event information and other data. The technology used this time, however, was developed through a combination of the AI technology that is one of Toshiba’s greatest strengths and the weather prediction technology Toshiba has developed through long years of research and development. Hiromasa Shin, Masaaki Takada and Yoshiaki Shiga of the Corporate R&D Center share their thoughts on the project.
From left to right: Hiromasa Shin, Masaaki Takada, and Yoshiaki Shiga
Toshiba Corporate R&D Center
“If the precision of the load forecast is low, the provider has to draw up a supply plan with some amount of leeway. This leads to standby operation and other wasted energy at the power plant. There have been attempts to introduce AI to fix such shortcomings, but operating and business flow issues made it impossible to fully automate the process. Forecasting always had to be done manually in the end. However, once the number of small enterprises increased, it meant a rise in the number of locations without enough manpower for forecasting. This increased the need for high-precision automatic forecasting using AI.” (Shin)
AI electrical load forecasting technology was far from practical use for a long time. What advances have occurred in the field now?
Using sparse modeling technology for data extraction was the key to increasing accuracy
“To explain in detail, this forecasting process uses AI to analyze future loads based on past data, weather predictions and event information. We then use the discrepancies between the predicted and actual values as machine learning feedback to improve the AI. What made the greatest difference is the fact that Toshiba’s proprietary weather forecasting data has grown greatly from the past in terms of both volume (number of measuring points) and variety (meteorological elements).” (Shin)
In the past, only 6-7 locations’ data of prefectural capitals were used in order to cover TEPCO’s supply area of around 40,000 square kilometers. This time, however, Toshiba team generated weather prediction values based on the 100 observation points of the Automated Meteorological Data Acquisition System (AMeDAS). They also utilized a machine learning technique known as sparse modeling to select the data from sites likely to have a high influence on electrical load from the weather information we had from multiple sites.
*1: Sparse modeling
A modeling technique that extracts meaningful data from a large amount of data.
Sparse modeling technology utilizes weather forecasting data from many sites
Automatically selects important sites that affect the load quantity (e.g. variations in important sites depending on the period of time)
The Toshiba team was formed when Shin sought out the expertise of Shiga, an expert in AI technology, and Takada, a sparse modeling professional, after receiving the notice of the contest.
“I had been working on using big data such as machining conditions and sensor values obtained during factory manufacturing processes to develop technology that could pinpoint the causes of declines in quality. I used sparse modeling to identify the true causes from among a massive list of potential factors, so I was really intrigued by the novel concept of applying the same technology to electrical load forecasting. Sparse modeling still has a lot of undiscovered potential, and I had already started thinking about deploying it in other fields besides the current business when I was asked to join the team, so the invitation came at exactly the right time.” (Takada)
What is ensemble learning and why was it crucial to success?
The Toshiba team next turned their attention to resolving the discrepancy between predicted values and actual results. For solar power generation in particular, the amount of power generated can vary depending on weather conditions and the load can vary minutely depending on human behavior, so massive calculation errors can occur.
The team feeds that massive error data back into the AI to create a repeated cycle of correction, and when they do, they use what is known as ensemble learning technology. “Ensemble” just means a group or a collection. There is a wide variety of AI methods, and the combination of these methods led to the accuracy of the predictions being improved.
“The method we used this time, which learns the regularities between the predicted and actual values, had its good points and drawbacks. For example, it would give accurate night-time predictions, but then it would be overly sensitive to day time temperatures, and so on and so forth. To help resolve that, we used an ensemble of AI technologies and blended them into the most precise combination we could manage after analyzing which time frame each technology performed best in.” (Shin)
Ensemble learning technologies blends data of deep learning predictions
As a result, they created a method that reduced the predictive error by 0.5-1.0% compared to conventional methods. A reduction of 1.0% in the predictive error is said to reduce power supply costs by about around 0.1%. So if, for example, the cost of supplying power per year was ¥1 trillion, that would mean potential savings of ¥1 billion per year.
Winning the grand prize in the contest proves the importance of this achievement. Looking back on the project, Yoshiaki Shiga, who supervised the whole system, commented:
“For this contest, it wasn’t enough to put the results of our past research and development into a system; we also had to link the system to the contest organizer’s system and complete everything within the allotted time frame. It was an extremely tight schedule, so I am really relieved that it all ended in success.” (Shiga)
Naturally the next goal is to make this technology available for practical use. The research team is working hard to adjust the system with the aim of making it available by 2020. Since load forecasting technology will be one of the invisible supporters of the society of the future, it would continue to receive the attention it deserves going forward.