Applying AI image recognition with society – Overcoming challenges with AI monitoring technology
2022/04/25 Toshiba Clip Team
- What are the three challenges that need to be overcome in order to implement AI with society?
- The results of the proof-of-concept test obtained from Toshiba’s image recognition AI and monitoring technologies.
- Toshiba’s current idea for preventing a conflict between technology and ethic.
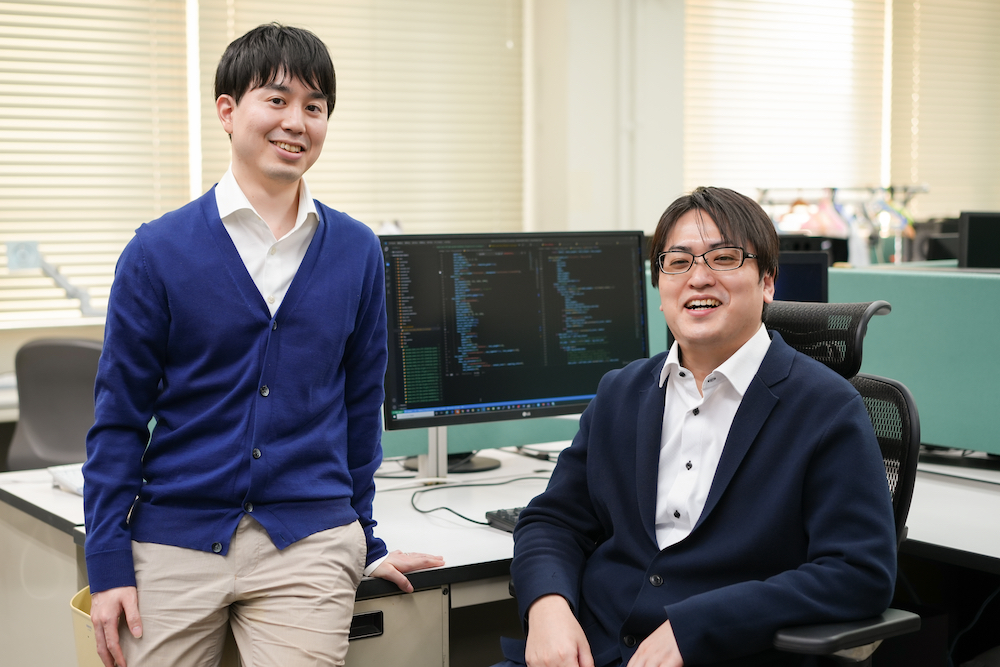
Robot vacuums are popular companions that make our lives more comfortable. They are equipped with artificial intelligence (AI) that can recognize objects, and are able to tell the difference between trash, cords, and other items. This is just one of the ways that AI is familiar to us, and that’s because we are keenly aware that they enrich our lives and we’re convinced that using AI is the right thing to do. Just how do cutting-edge AI technicians perceive this keen awareness and convincement? What are they working on? What sort of future do they envisage? Tomoyuki Shibata and Daisuke Kobayashi of the Corporate Research and Development Center, Toshiba Corporation, speak about the case of an image recognition AI proof-of-concept test that measures things such as the level of crowding at a cafeteria.
The three challenges to overcome in order to implement AI with society
First, let’s take a look at the outline of the proof-of-concept test. It was conducted from July 15 to 30, 2021, at a Toshiba’s cafeteria. The test sought to verify whether the image recognition AI technology developed by Shibata and Kobayashi detected cafeteria users accurately – specifically the flow of people, degree of crowding, and other factors.
At that time, Shibata and Kobayashi turned their eyes to Machine Learning Operations (MLOps), another technology under development by the same Corporate Research & Development Center. MLOps is a platform that runs the AI operation and learning cycle. By putting the developed AI on top of this platform, it is possible to continually maintain and improve its performance.
How the MLOps flow works are…. (1) The AI is operated on the MLOps platform, (2) MLOps continuously monitors the AI and checks if it is functioning as expected. If there is a deviation from what is expected, (3) re-train the AI based on the data, customize it to the site, and return to (1). Furthermore, (4) data obtained from operations and past AI models will be accumulated in the data infrastructure and utilized for the next AI development. It’s MLOps that automatically runs this cycle. By operating AI via this platform, technicians such as Shibata and Kobayashi are able to efficiently verify the accuracy of AI, enabling it to be implemented on site. (For more details on MLOps, see AI is a journey, not a destination – Toshiba’s AI progresses to the next stage)
How Machine Learning Operations (MLOps) automatically and continually maintains and improves AI performance
“Toshiba’s image recognition AI has already met a certain technical level. Right now, we’re at the stage of making people keenly aware that AI is a meaningful presence. In order to do this, we need to be able to efficiently check whether AI is functioning as expected on sites, and continually maintain and improve its accuracy.
And when there is an anomaly, it’s important to be able to explain the reason—whether it’s due to the AI or due to data. This helps convince the people who use AI. In other words, we AI technicians aren’t aiming to implement AI in society. We’re aiming to implement it with society. That’s why we used MLOps,” says Shibata.
Tomoyuki Shibata, Expert, Media AI Laboratory, Advanced Intelligent Systems Laboratories,
Corporate Research & Development Center, Toshiba Corporation
That’s what Shibata has to say about the background to them using MLOps. When we asked him a few more questions, Shibata explained that “there are three challenges to overcome in order for image recognition AI to be broadly accepted by society.”
The first is ensuring the quality of AI. There is a limit to the amount of data that can be used when developing AI. So, in order to achieve the performance expected by users, AI may need to learn from the data in the environment after it has been introduced to a site. As was mentioned earlier, if the AI is operated on MLOps, it will be operated to fit the needs of each site.
The second is speeding up the process from AI development to testing and introduction. The COVID-19 pandemic has given rise to more situations where AI is needed, such as for measuring the density of people. Up until now, technicians who developed the AI would go on site, run tests, and proceed with implementation. However, if the AI developed is run on MLOps, verification can proceed without the need for human intervention. This means that AI can be introduced faster and be used in a wider variety of situations.
The third is raising the explainability of AI. When image recognition AI has produced and reported unexpected results, we will feel anxious if there is no indication of what went wrong. If MLOps is operated with AI, monitoring will indicate the reasons for abnormalities, and so, we can take appropriate countermeasures. This makes the answer provided by the AI more convincing, and AI will be more likely to be accepted by society.
Toward the social implementation of AI: a solid response obtained through the test
So, just how will Shibata and his team overcome these three challenges? Let’s revisit the test in the staff cafeteria and hear what Kobayashi has to say about the results.
Daisuke Kobayashi, Specialist, Media AI Laboratory, Advanced Intelligent Systems Laboratories,
Corporate Research & Development Center, Toshiba Corporation
“Even though the proof-of-concept test was carried out over a limited period, we were still able to get the results as we expected. More specifically, when we ran the image recognition AI on MLOps, the monitoring function correctly judged an abnormality in the noise we intentionally made, such as obstacles placed in front of the camera or backlighting. On the contrary, noise which should not impose a problem for image recognition AI was judged to have no abnormality by the monitoring function,” says Kobayashi.
Kobayashi mixed around 60% of noise such as obstacles in the video data to be processed by the image recognition AI. This time, the monitoring function that watches the behavior of the image recognition AI judged that there was an abnormality only for the noise. Furthermore, the remaining 40% of the data, which does not affect image recognition AI output and cannot be called noise, was judged to have no abnormality. We were able to determine that there was an abnormality only when there was noise, and no abnormality only when there was no noise, thus clearing the first challenge, ensuring quality.
Monitoring run by MLOps judged the presence of noise with 100% accuracy
So, how about the second challenge, “speeding up AI testing and introduction,” and the third challenge, “raising the explainability of AI”? Kobayashi felt that MLOps monitors AI at a level comparable to that of him to go around the site and testing. “If testing continues at this level, we can implement AI without any problems.” he says. This means that it will be possible to introduce AI on site without the need for an AI technician to visit the site. Kobayashi also shared an anecdote relating to the explainability of AI.
“When we first started running image recognition AI on MLOps, one day it gave an alert that there had been an abnormality with the video. When we asked the people working at the cafeteria if something had happened, they told us that it had been so busy and staff had not been able to eat their staff meals on that day, and their movements had been different to usual. Everyone was quite convinced by the AI’s judgement,” says Kobayashi.
Toward a world where technology and ethics are in harmony
The use of MLOps has boosted prospects for fostering a “keen awareness” and “convincement” about the image recognition AI developed by Shibata and Kobayashi. Shibata spoke about his hopes, saying “MLOps will enable testing, introduction, and popularization of image recognition AI to progress efficiently, and to gain more users. It can contribute to the realization of an abundant society.”
New projects are already underway. For example, one new need that has arisen due to office reforms and work-style reforms during and after the COVID-19 pandemic is visualization of communication. If image recognition AI can shed light on which interactions are meaningful and how to discuss effectively, this will boost well-being in the sense of work satisfaction. In addition, discussions with major retailers have focused not only on monitoring for safety and security, but also on improving the efficiency of cleaning, dealing with lost children, and assisting with purchasing, given the labor shortages. In each of these cases, the accuracy of the image recognition and the keen awareness and convincement of the user are vital. That’s why it’s so meaningful that this proof-of-concept test has overcome challenges.
At the same time, there’s one thing that Toshiba must always remember. There are two key issues that need to be addressed when implementing AI with society: ethical considerations such as privacy, and consumers’ comprehension. Shibata told us about the latest initiatives.
“Toshiba is working on a joint project with a national university to study social norms, ethics and governance for the use of image recognition AI in public spaces. While image recognition AI is vital for a safe, secure, and abundant daily life, it’s important to avoid provoking anxiety through proper operation. We are now carefully interviewing local residents to identify and verify the challenges that need to be addressed before society accepts image recognition AI,” says Shibata.
Even if an image recognition AI has outstanding capabilities, that doesn’t mean that society will accept it as-is. We need to consider the possible risks and keep an eye on the governance to ensure that AI is used effectively. In order to avoid conflict between technology and ethics, there is no doubt that the image recognition AI developed by Toshiba will further evolve and deepen by operating it with MLOps.
Related Links
*This section contains links to websites operated by companies and organizations other than Toshiba Corporation.
Toshiba AI Technology Catalog | Toshiba