Creative Thinking Realizes a New Normal and the World’s Most Accurate AI, Part2 -Reducing AI training data while improving performance
2023/08/23 Toshiba Clip Team
- Bringing AI and digital transformation to companies with fewer data resources.
- An innovative approach solves the problem of abnormality over-detection.
- Bringing 50 years of experience and expert talent to AI development.
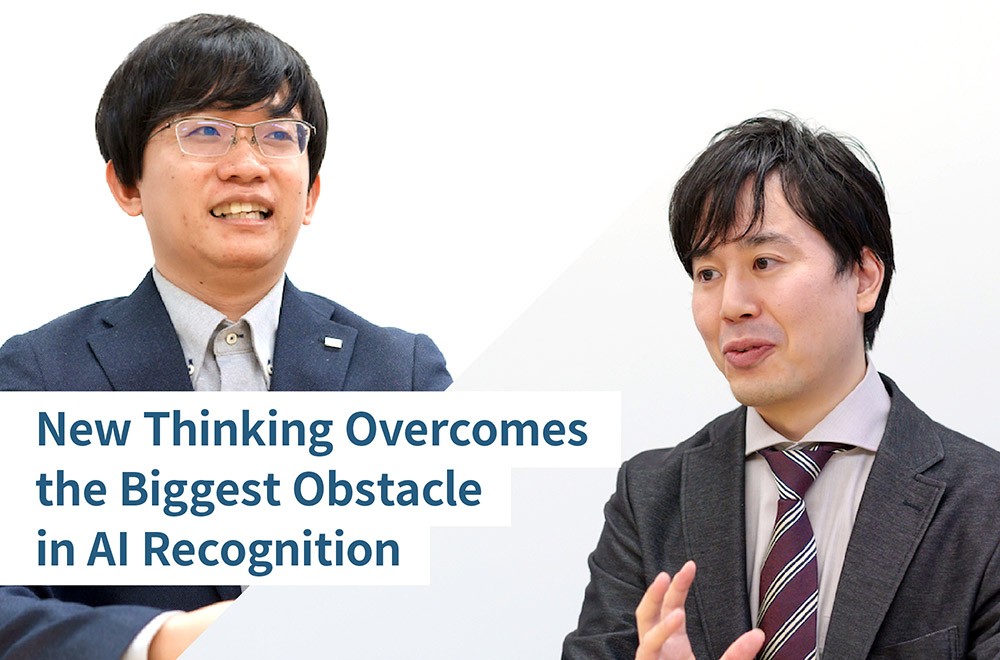
AI-based image recognition is already part of our lives. What is not often realized is that to get it to work with a high degree of accuracy requires extensive training with large volumes of data. This is a problem that is holding back even wider application, but Toshiba is pioneering two solutions, Few-shot Object Detection AI and Abnormality Detection AI. Not only do they reduce training requirements, they are also the world’s most accurate AI models*1.
*1: Toshiba research as of February 2022
In Part 1 we looked at the features of these advances, and some of the ways they can contribute to society. Here we take a closer look at the development of the AI—the breakthroughs in R&D—and the value they bring to the world. Once again, we spoke to the people behind the innovations about their work, and also asked them how Toshiba’s 50 years of experience in AI research add up to unique strengths.
Bringing digital transformation to companies with limited data resources
Toshiba numbers among the world top ten companies in AI patent filings, the outcome of over 50 years of research and development that have generated expertise in many areas, among them speech and language recognition, image recognition and data analysis. A major breakthrough in image recognition came as long ago as 1967, with machine recognition of handwritten characters and numbers. The successor to this technology is still in use today, in automated post code reading and letter sorting machine.
Naoki Kawamura, the developer of the AI for detecting abnormalities in social infrastructure says that. “Our strengths in facial recognition and object detection rest on decades of cumulative expertise. This also gives us an advantage in making the most of our human resources.”
Naoki Kawamura,
Media AI Laboratory, Advanced Intelligent Systems Laboratories,
Corporate Research & Development Center, Toshiba Corporation
To find out how Toshiba’s expertise and talent enabled development of the world’s most accurate image recognition AI, models that do not rely on large amounts of data, we took a deeper look at the Abnormality Detection AI and Few-shot Object Detection AI.
Whatever the area, R&D at Toshiba is characterized by close attention to customer needs and challenges. In the case of the Few-shot Object Detection AI, as so many times before, it was customer feedback that triggered the project. Daisuke Kobayashi reflected on the lead-up.
“We had customers who wanted to use AI to identify unknown objects. They wanted to streamline operations by using image recognition AI to convert on-site conditions into digital data, with the ultimate goal of digital transformation (DX), but they could not afford the time or cost of amassing the training data needed to develop the AI. Our solution to this conflict was the Few-shot Object Detection AI, which can learn even with small data sets.”
Daisuke Kobayashi, Specialist,
Media AI Laboratory, Advanced Intelligent Systems Laboratories,
Corporate Research & Development Center, Toshiba Corporation
Few-shot Object Detection AI is twice as accurate as conventional detection methods
Developing an AI image recognition model with minimal data is much more easily said than done; some people even said it was impossible. It turned out that the most important factor in its achievement was a new way of thinking about how AI could be trained to recognize images.
“I was chatting with a colleague working on another project about what way of thinking could help us overcome challenges,” recalls Kobayashi, “And in the middle of it, this idea came to me. Once I got my head around it, I saw how to organize my thinking, and the work went smoothly from there.
“To be specific, we changed the AI mechanism. Instead of just looking for objects tagged in the training data, we trained it to see things that were previously treated as background as objects for detection. This gave us a significant improvement in detection accuracy, taking it from 21.2% to 46%.”
Few-shot Object Detection AI detects all object candidates and matches them with the target image
With this new method, the AI stopped looking for the one correct answer and instead started to identify “correct-looking” objects. These were then compared to the actual target object for detection to identify the right one. This approach had a huge impact, as Kobayashi explains: “When the AI is used in factories and plants that handle a lot of different parts and equipment, it can detect target objects without the need to prepare the large amounts of data that were required until now. I think this gives companies that have been unable to capture onsite conditions digitally a real opportunity to create value through DX.”
The Abnormality Detection AI was developed through reverse thinking
Kawamura, the developer of the Abnormality Detection AI, also starts his story with an issue facing a customer. Toshiba has long been involved in building social infrastructure, and was consulted by one of its main customers, a major electric power company, about a problem they had to deal with during inspections. It intrigued Kawamura as an AI developer.
“Outdoor infrastructure is installed in many different environments, and exposed to wind and rain, all sorts of conditions. They are also installed across vast areas that take time and a lot of effort to inspect, and there are sites that need to be inspected but that are difficult to access. Drones and helicopters are used to inspect some of these inaccessible locations, but the photos they take do not always reveal abnormalities.
“The public relies on social infrastructure, and it is designed and built to be resilient, with little room for error. As a result, inspections often do not come up with the abnormality data needed for training. Our customer had this problem, and wondered if we could come up with an AI that could find abnormalities with only a few photos, or from images that were off-center or unclear. That is how the project started.”
The idea of abnormality detection AI was not a new one. It had already been investigated in numerous papers, but all of them reported a major problem: over-detection, where even normal parts were determined to be abnormal. Over-detection was also the main obstacle in Kawamura’s project, and when earlier AI determined that normal parts were abnormal the technology had been abandoned, because of what was seen as a technical failing. Kawamura, however, took a different approach, a reversal of thinking that stemmed from expertise gained from his earlier work on AI facial recognition: “If the AI is over-detecting, why not simply subtract that from the results?”
As Kawamura explains, “AI facial recognition has to distinguish between people who look similar. It recognizes individuals by referencing hundreds of feature points, and misrecognition of one person as another is due to them having similar features. That understanding gave me the idea of developing an AI that ignores those similar areas. That’s the knowledge we brought to this project.
“When AI is applied to social infrastructure, the normal images in the training data naturally have different brightness levels, and there are shadows and other artifacts. These can confuse the AI, which labels them as abnormal. We worked out a formula to reduce false detection of abnormalities, and this produced a recognition accuracy of 91.7%, the highest level yet recorded.”
Appropriate detection of abnormal parts by suppressing over-detection in “normal” images
Potential applications include transmission towers in mountains, bridge deck undersides, and solar panels
How will the Abnormality Detection AI be utilized? Here’s one example: Dash cams and drones capture images of objects in all kinds of positions and orientations. As many of them are taken at odd angles or are off center, AI classifies most of them as abnormal, making it close to useless. Once the Abnormality Detection AI is put to use, it should be able to work with data from dash cams and drones.
The development objective behind the new model is that users need only a few images of an object in its normal state, taken during mandated inspections and the like. By comparing these images with those from drones and other sources, abnormalities can be promptly detected. There is great potential for sites where unmanned labor-saving inspections are required, such as transmission towers in mountains, the underside of bridges decs, and the backs of solar panels.
“I don’t think application is limited to social infrastructure,” says Kawamura. It can be used for farms and other places where there are large numbers of similar structures in a row, as its easy to get ‘normal’ patterns. While we are still in the research phase, the AI should eventually be useful in a lot of situations where there are images of things have to be compared. We will work with a range of customers, including distributors, to improve the technology through repeated demonstrations and tests.”
Toshiba’s AI is backed by decades of history and deep talent
As noted, both new AI models are technologies that have been studied in many papers. However, Toshiba has gone a step further by working on development for use in the field. The ability to do this lies in Toshiba’s attitude to AI and its cumulative history.
Kobayashi describes Toshiba’s research labs as “a group of specialty stores;” their raison d’être is technologies that are used by internal business units and outside customers.
“The research labs are connected to our business units and are always on top of issues in the field. Of course, we also receive feedback on how our technology is actually being used. We identify what kind of technology is lacking in the field and conduct research and development on how to make technology that is easier to use.
“Many technologies end up not being implemented, and the accumulation of these bitter experiences—technologies lauded on paper but never used in real life due to constraints in the field—has brought us to this point. We constantly ask ourselves, ‘How do we get customers to use our technologies successfully?’ This is what motivates us. I feel the greatest sense of accomplishment when people in the field are happy with what we have developed.”
Commenting on the quality of Toshiba’s people, Kobayashi and Kawamura agree that “researchers feel very much at home at the R&D Center.” While the technologies covered in these articles clearly reflect the talent of their creators, but there can be no doubt that discussions between experts in the labs (shops) were an important background.
The development team behind the Few-shot Object Detection AI and Abnormality Detection AI. An environment conducive to discussion breeds innovative ideas.
“Toshiba’s labs are full of talented people more than capable of university-level research centers,” says Kawamura. “While they research different things, when they are stuck and looking for some inspiration they can discuss things with one another, and this contributes to one breakthrough after another. With so many high-level engineers in one place, discussions are fun and lead to new ideas.
“In developing the Abnormality Detection AI, we managed to propose a technology that could be used in fields we had not been involved in before. We will continue to take on challenges in various fields and contribute to all kinds of businesses with easy-to-use technologies.”
“Both Kawamura and I were entrusted with R&D responsibilities from our first year at the company,” notes Kobayashi. “As long as you’re passionate about your work and have honest opinions, it’s an environment that empowers you to take on challenges regardless of your age.
“Alongside accuracy, we constantly think about where AI can be used in the social system. We will continue to create user-friendly technologies by listening to feedback and identifying social issues.”
Few-shot Object Detection AI and Abnormality Detection AI turn a new page in Toshiba’s long history of AI development. The exceptional talent and depth of the people involved in AI at Toshiba, and the real-world orientation of the research environment that nurtured their talent, paved the way for the creation of technologies that detect objects and abnormalities with world-class accuracy but only a small number of images. This same foundation will underpin Toshiba’s development of new AI technologies and drive the promotion of DX in society.
Related Links
*This section contains links to websites operated by companies and organizations other than Toshiba Corporation.
Toshiba AI Technology Catalog | Toshiba