How Do You Ensure the Quality of AI Systems?, Part 2 -Our mission to improve AI literacy
2023/03/23 Toshiba Clip Team
- The key to AI quality is the quantity and quality of training data.
- High quality is a prerequisite for AI systems.
- Design for safety, knowing that AI can make mistakes.
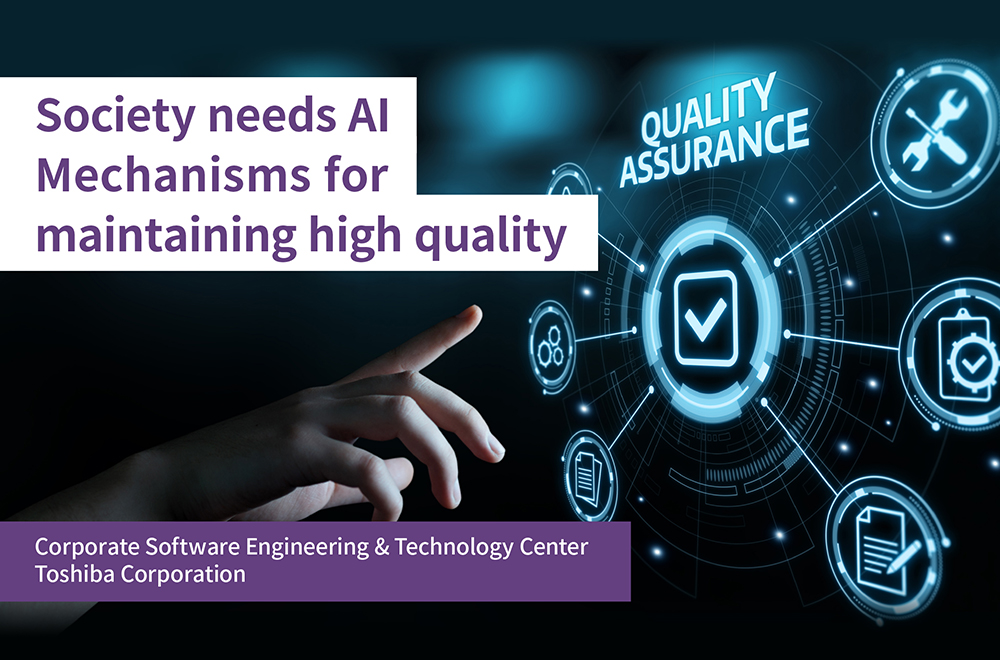
The phrase “garbage in, garbage out,” GIGO, is a useful reminder that AI systems trained on low quality data will pick up unwanted features, resulting in poorly realized AI models.
In Part 1, we looked why AI quality is now attracting so much attention. Europe has already started to enact laws and regulations on AI quality, while Japanese companies and research institutes are publishing their own guidelines in an effort to keep up with current trends. This article introduces how Toshiba, a leading AI company, is tackling this challenge. Commentary is once again provided by Kei Kureishi, leader of the AI Quality Assurance Project at Toshiba’s Corporate Software Engineering & Technology Center.
Machine Learning Engineering: The creation of a new academic discipline
When the realization of technologies for machine learning and big data triggered the third AI boom—the quest for data-driven, practical AI products—AI was initially confined to the research labs of academia and industry, far from real-world implementation. However, once systems went beyond the lab setting and use by a limited number of people, and started to appear as products and services, problems emerged for society. A typical issue is bias, where often unconscious assumptions in input data influence results.
Kei Kureishi, Expert, Software Engineering Technology Department,
Corporate Software Engineering & Technology Center, Toshiba Corporation
“Machine Learning Engineering, an academic discipline, was developed to solve these AI-related issues,” Kureishi explains. “In 2018, the Japan Society for Software Science and Technology established a Special Interest Group on Machine Learning Systems Engineering, to study best practices and develop systematic approaches for integrating AI into systems.”
Since around that time, ensuring the quality of AI systems has become an important global issue. Japan’s Consortium of Quality Assurance for Artificial-Intelligence-based products and services (QA4AI Consortium), of which Kureishi is a member, has published the QA4AI Guidelines, and other groups in Japan are independently promoting quality initiatives that reflect their own perspectives—for instance, The National Institute of Advanced Industrial Science and Technology (AIST), has released Machine Learning Quality Management Guideline. In Europe, the European Commission announced a proposal to regulate artificial intelligence* in 2021.
* “Proposal for a Regulation of the European Parliament and of the Council Laying Down Harmonised Rules on Artificial Intelligence (Artificial Intelligence Act) and Amending Certain Union Legislative Acts.”
Commenting on the different approaches in Japan and Europe, Kureishi said, “The policy in Europe is to legally regulate new technologies as they emerge. In Japan it is unusual to enact legislation so quickly. At the moment, the mainstream policy here is to set rules and tackle them within companies or industries.”
The key to AI quality is data quantity and quality
AI is built on data, and its quality is determined by the quality of the training data. Simply explained, an AI builds a model from past data and uses it to make decisions on new data. The problem is, however, that if the training data is insufficient in quantity, the AI will make wrong decisions, with no way to improve its accuracy. Here, quality is synonymous with quantity.
The fact that demand for AI cannot be met unless data is available in sufficient quantities raises a question now being addressed around the world: how to secure the necessary data? Some countries scoop up data on a large scale, from the footage of cameras installed in cities, for example. But that cannot be done in Japan and other countries where privacy is a concern, which has prompted many researchers, looking at things from the perspective of innovation, to worry that differences in data collection will impact AI development.
Alongside quantity, the condition of the data, its quality, is also crucial. No matter the volume, data is not useful if it only partially represents the phenomena to be captured—it makes no sense to train an AI system on data of a specific age group if it has to predict the behavior of the entire customer base. So the question of quality also extends to the kind of data collected.
To maintain quality, keep updating the training data
Shifting perspective a little shows that time is also critical factor for AI quality. As noted, AI uses historical data to build its model, and naturally enough, accurately predicting the kind of data it will have to deal with once it has been rolled out is impossible. As the world changes so too does the data it generates. This raises the question of how accurately AI can use past data to make predictions about the future.
Toshiba’s response is Machine Learning Operations (MLOps), a mechanism that enables an AI system to relearn with the latest data, even while in operation. MLOps monitors the status of AI systems, operating in various environments, and if something unexpected happens it makes the AI relearn from the new data. With MLOps, an AI model can automatically adjust to changing realities with course corrections that maintain and improve its performance.
How MLOps maintains and improves AI performance
AI is now used in a wide range of products and services. Toshiba’s AI models are primarily used in social infrastructure, such as transportation and electric power systems. This requires a unique approach to AI quality, as Kureishi explains.
“AI for use in social infrastructure must deliver stable operation over a long period. If it were to stop operating or malfunction, it would have a negative impact. At Toshiba, the need for high quality is a given, and we achieve the accuracy required for social infrastructure applications by evaluating and developing reliable AI models that balances performance with stability.”
Technologies that ensure the high quality of Toshiba’s AI systems
Over the 100 years and more that Toshiba has contributed to and supported social infrastructure, it has amassed knowledge and expertise and handed it down through the generations. AI technologies derived from this knowledge base cover quality visualization, testing, and quality evaluation.
Toshiba refines its quality visualization, testing, and quality evaluation techniques
based on the Quality Assurance Guidelines for AI Systems
During the implementation of an AI system, quality visualization makes it easier for Toshiba’s non-expert clients to understand the workings of the system. This technique is important for maintaining and improving the quality of the AI, because non-experts must accurately understand its characteristics and be able to use it. A standard card format is used to summarize the characteristics of the data used in training and the development of the AI model, and to visualize the results the AI generates both qualitatively and quantitatively. Applying the technique helps clients to understand the AI on a sensory level, instinctively.
Testing confirms that an AI meets the required specifications. Unlike traditional software, AI systems do not follow blueprints and require the construction of unique testing methods specific to the AI and its training data and model.
Quality evaluation focuses on data quality and model quality. It verifies that data used to train the AI has the required scope and coverage, and whether or not the AI has the robustness to operate properly when fed data other than that provided for training. This is an area where Toshiba is promoting rapid progress in developing concrete test methods and evaluation techniques.
Designing for safety with the awareness that AI makes mistakes
As we have seen, AI quality is significant in its own right and also a critical requirement that impacts on utilization and results. Imagine the use of AI in diagnostic medical imaging. Here quality means a high accuracy rate, and that is clearly important. However, since healthcare is concerned with human lives, safety is an equally important consideration, and design of the AI must be done on the premise that AI makes mistakes.
In some cases, systems are programmed to stop in mid diagnosis and the final judgement is left to a physician. Another way to utilize AI is to combine AI and human judgment, for example, in self-driving cars, where AI decision-making is suspended under certain circumstances and the option of driver operation offered.
“Our approach is that quality is a prerequisite, not a matter of being competitive,” says Kureishi. “The idea is not that high quality sells, but that quality must be guaranteed in order for us to sell it. From this, we believe it is necessary to improve quality by sharing information among all involved parties. This is perspective that led us to present our quality visualization technique to the Special Interest Group on Machine Learning Systems Engineering. It received a lot of attention, and we felt that there was a great need for such a technique.
“Quality in AI is similar to the concept of security. It’s obvious that it needs to be upheld, and the way that is done makes a difference. I think quality in AI is a matter of sharing where the threats are and improving quality across society.”
If we are to use AI to help realize a better world, we must ensure the quality of AI systems. We must make sure that the AI we build into systems does not cause disappointment or result in negative reactions. Only then will AI be seen as a positive thing and more widely used—the essence of Toshiba’s wish and mission.
“Although I am not directly involved in developing AI, I do work behind the scenes in quality assurance. I want to support our mission from a quality perspective,” Kureishi says with conviction.
Related Links
*This section contains links to websites operated by companies and organizations other than Toshiba Corporation.
TOSHIBA REVIEW: SCIENCE AND TECHNOLOGY HIGHLIGHTS 2022; Research and Development