Creative Thinking Realizes a New Normal and the World’s Most Accurate AI, Part 1 -AI Image Recognition No Longer Needs Huge Volumes of Training Data
2023/08/09 Toshiba Clip Team
- “Data hungry.” An issue for use of AI in manufacturing and infrastructure.
- Toshiba’s object detection AI needs only a few images—sometimes just one.
- Toshiba’s Abnormality Detection AI correctly detects a wide range of abnormalities with minimal input.
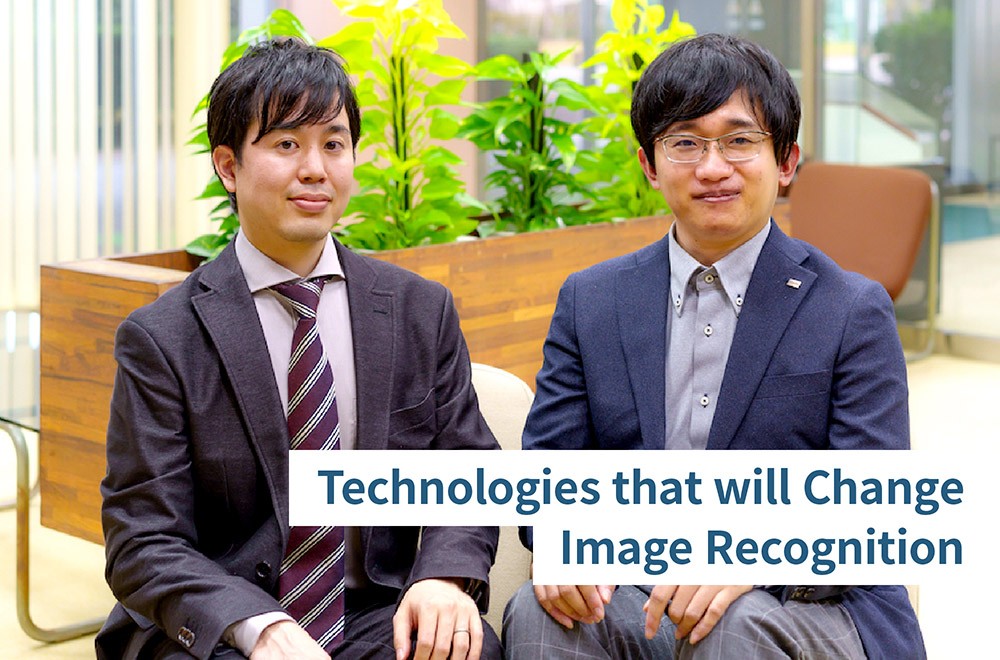
The market for AI is expanding rapidly in Japan. Some predict that by 2025, it will swell to 1.9 trillion yen, about double the FY 2020 level, as AI is increasingly used to solve social issues. In March 2022, IRCAI, UNESCO’s AI research organization published a list of 100 projects that mobilize AI to achieve the Sustainable Development Goals (SDGs). For example, mapping using AI and satellite imagery to create an accurate map of school locations was highlighted as a solution addressing the issue of lost educational opportunities due to a lack of records of school locations.*1 AI image recognition, the focus of this article, is likely to be adopted in a variety of industries and types of business as its accuracy improves through deep learning, and there are high expectations for how the results of such AI analysis can be utilized.
*1: School mapping using AI and high-resolution satellite imagery
Highly accurate AI image recognition models, like the one to analyze satellite images mentioned above, must be trained with huge volumes of image data. But collecting that data is not easy or cheap, and is a high development hurdle. Toshiba has solved this problem with two models that require much less image data, but are the world’s most accurate*2 in their respective categories: Few-shot Object Detection AI and Abnormality Detection AI. In Part 1 of this article, we talk with the developers and summarize the key points of each AI. We also look at their significance within the current state of AI image recognition.
*2: Toshiba research as of February 2022
“Data hungry.” An issue for use of AI in manufacturing and infrastructure.
Around 540 million years ago, at the start of the Cambrian Period, many new and remarkable lifeforms suddenly emerged. Biologists group organisms with the same body plan in phyla, and the most striking thing is that most phyla we see around us today, much of the diversity of life on earth, appeared at this time. Zoologist Andrew Parker has explained this with the Light Switch Theory, which postulates that the emergence of organisms with eyes was a key factor in explosive evolution. Commentators on AI often liken the recent potentially world-changing advances in machine learning, the ability to see and analyze huge volumes of data, to the emergence of eyes; and one area where this is true, and truly important, is image recognition.
That deep learning has contributed significantly to the development of AI image recognition is indisputable. But it would be a mistake to think that deep learning is a magic wand that can do anything with a flick of the wrist. Daisuke Kobayashi from Toshiba’s Corporate Research & Development Center describes the challenges of AI image recognition as follows: “Image recognition AI must be trained to accurately identify objects, and the way this is done is with large volumes of data, training data. The more data you have, and the more correct information on what it represents, the better the deep learning process, and the more accurate the image recognition. However, putting together huge amounts of data costs time and money, and there are limits to what we can source from outside the company due to confidentiality concerns.”
Daisuke Kobayashi, Specialist,
Media AI Laboratory, Advanced Intelligent Systems Laboratories,
Corporate Research & Development Center, Toshiba Corporation
This huge appetite for data means that deep learning, including image recognition AI that use the technology, is “data hungry,” something definitely not liked in manufacturing and social infrastructure. Naoki Kawamura, a colleague of Kobayashi’s, explains why: “When a factory launches a new product, the production process often needs new equipment and materials. If the factory already uses AI image recognition to manufacture other products, it will need to add the new product for analysis. But training and retraining the AI with image data every time a new product is introduced is really expensive and takes time.
And that is only one concern. Equally important, according to Kawamura, is data frequency: “Social infrastructure, such as electric power infrastructure, is designed on the basic premise of stable operation. Even if we wanted to use AI image recognition to detect an abnormality, it would be rare to have the data on abnormalities the AI needs to detect. In other words, if we cannot collect the necessary abnormality image data, the AI cannot learn.”
Naoki Kawamura,
Media AI Laboratory, Advanced Intelligent Systems Laboratories,
Corporate Research & Development Center, Toshiba Corporation
Toshiba’s Object Detection AI can accurately recognize objects—even from a single image!
Aware that data-hungry methods and concerns about data frequency are discouraging many companies from adopting AI, Toshiba looked for solutions. The outcome is two new models: Few-shot Object Detection AI, developed by Kobayashi, and Abnormality Detection AI, developed by Kawamura. Both do more than significantly reduce data requirements, they are also the world’s most accurate models in their respective categories, able to detect previously unseen or studied objects and abnormalities with high levels of accuracy.
Kobayashi describes the Few-shot Object Detection AI as, “Technology that detects a new object using one to ten images and corresponding correct information.” The theory on which this AI is based is not unique to Toshiba; it is well established and being researched by others. So what exactly is new about Toshiba’s Few-shot Object Detection AI?
“Conventional AI is trained to recognize and search for specific objects,” says Kobayashi. “This is a bird, correct; that is not a bird, wrong, and so it is relegated to the background and ignored. Toshiba’s model is first of all trained to looks for objects in an image, any and all objects: that’s an object, that’s an object, and that’s an object, too.” Nothing is pushed into the background, and all objects are there to be detected.
What is happening here is that the AI is not concerned about what is correct or the names of objects, it is simply learning. It is absorbing heaps of image data from all sorts of sources, using them to create an algorithm and to make rules for how to recognize objects. It’s a process called self-supervised learning.
An AI model that recognizes all objects as objects
Once the AI can detect objects in general, it is trained to recognize specific objects, and they will change with where the model is used. Let’s say it is used in a factory, and we want it to detect screws. We simply train it with images of a screw, up to ten of them. That’s all it takes. Show it other images, and it will identify not only the object-like areas, but any shapes in it that are similar to a screw. Screws might all look different, but the AI can match images, categorize, and then tell us, look, here’s a screw. This may well be how kids learn as they grow, a similarity that is a major driving force for the adoption of AI.
“What was amazing was how the AI could autonomously recognize images of birds,” says Kobayashi. “I tagged only one image of a black, thin-bodied bird as a bird, but the AI also correctly identified a round, brown bird with fluffy feathers as a bird. Conventional AI tagged it a sheep, so this new method showed very high accuracy. I was surprised at the accuracy of the AI’s guesses, because the two birds were so different in type and appearance.”
Few-shot Object Detection AI accurately detects objects in images despite differing in type and appearance
Abnormality Detection AI overlays sample images, like transparencies, to make comparisons
Now, let’s explain the other AI image recognition model, Abnormality Detection AI. This AI is characterized by its ability to detect abnormalities with the world’s highest accuracy when inspecting bridges and other social infrastructure, using only a few images of normal conditions for comparison. In addition to relatively common cracks and rusting, less frequent abnormalities can also be detected with high accuracy: water leaks, adhesion of foreign matter, and fallen parts.
According to Kawamura, “We upload the image data we want to examine into the Abnormality Detection AI and compare this against normal image data samples. The system detects areas that look different from normal, and identifies them as areas with a high possibility of abnormality.”
Here’s how it works. The AI does have to be trained, and that is done with relatively accessible image data of normal social infrastructure, not vast amounts of data covering all sorts of anomalies. This creates a model that detects variations from normal, and that also eliminates excessive detection. This latter training stops the AI from zealously detecting things like slight shadows in normal image as problems; from advance comparisons of normal image data, the AI learns such differences can be ignored, and suppresses their detection. It learns that only areas that really demand attentions should be treated as abnormalities.
Suppressing over-detection by comparing normal images
With this training done, the model is ready to make assessments. Let’s say of a steel tower on a social infrastructure site. Photos of the tower in good condition are uploaded, and then photographs of its current condition. The Abnormality Detection AI aligns the images to get the same angle and position, and superimposes the latest photographs over the earlier one, like transparencies. Any deviations from the earlier images are tagged. They are assigned scores, and the photographs are colored to reflect the scores: red for higher levels of abnormality, blue for lower. The status of the tower is determined quickly, with just a few images and a high level of accuracy.
The AI detects abnormalities from several normal images and turns these areas red
“There are so many different cases of abnormalities that it’s simply impossible to keep count and detect them all,” says Kawamura. “When we used AI to identify these problems, we had to upload a huge number of images of cracks, of rust and leaks and the like. The development of this AI to detect abnormalities is a real breakthrough, as we no longer have to prepare large numbers of photos of the site.”
The Few-shot Object Detection AI and the Abnormality Detection AI are a record of major progress in reducing training data requirements and accurately recognizing objects after training with a small number of images. They hold out the promise of many operational applications. Although the concepts underlying both were already known and studied, the noteworthy thing here is progress toward actual implementation. Part 2 looks at why Toshiba was first in the world to realize AI models that can recognize objects based on fewer images. We will take a closer look at the story behind the research and development that went into these models, and at Toshiba’s vision for the future.
Related Links
*This section contains links to websites operated by companies and organizations other than Toshiba Corporation.
Toshiba AI Technology Catalog | Toshiba